News
Faculty Spotlight: Joydeep Biswas
Joydeep Biswas is a core faculty member at Texas Robotics leading research on long-term autonomy through his research group, the Autonomous Mobile Robotics Laboratory (AMRL)
AMRL's focus is working to enable robots to navigate and reason in unstructured human environments over extended periods of time.. Recently, AMRL's research culminated in an autonomous deployment on a trail through Eastwoods Park in Austin, Texas. The robot was able to navigate the terrain autonomously, using the insights it had learned from human demonstrations and its own observations.
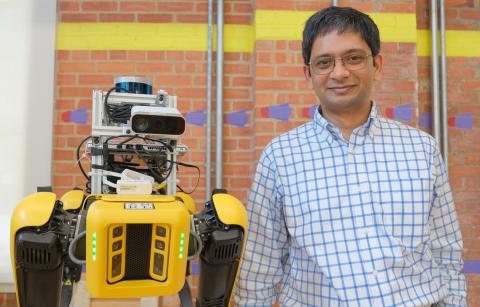
Joydeep Biswas, a core faculty member at Texas Robotics since Fall 2019, is leading groundbreaking research for his lab, the Autonomous Mobile Robotics Laboratory (AMRL) in support of long-term urban-scale autonomy. The focus of one recent project in his lab is on terrain adaptive navigation, a field that aims to enable robots to navigate and reason about different terrains in the same way humans do.
Traditionally, robots have been pre-programmed with visual models to identify specific types of terrains, and situational examples for specific environments, but this approach is not scalable or adaptable for new situations. Joydeep's lab is taking a different approach through teaching robots to learn from a combination of their own observations and human demonstrations to make autonomous decisions.
One of the key challenges in developing terrain adaptive navigation systems is the need for robots to visually distinguish key elements in the environment and human preferences in relation to them. For example, a robot must be able to learn that walking on a sidewalk is better than walking on grass, and how to visually differentiate them from a distance. To overcome this issue, one recent approach from Joydeep’s lab, titled visual representation learning for preference-aware planning (VRL-PAP), has a human show a robot the desired path to take. The robot then gathers information on both the demonstrated path and other potential paths that the robot considered taking, but the human avoided. By comparing the differences between the demonstrated path and other potential paths, the robot can learn to visually distinguish the different types of terrain, and which differences are more impactful and important for future decision making.
This research allowed the robot to autonomously follow a trail through Eastwoods Park in Austin, Texas. During this deployment, the robot was able to navigate the terrain autonomously, using the insights it had learned from human demonstrations and its own observations. This demonstration is a significant milestone in the development of terrain adaptive navigation systems - it demonstrates that approaches like VRL-PAP are indeed effective at teaching robots how to reason about path preferences and to visually identify terrains.
Looking to the future, Joydeep and his team are exploring the potential for robots to learn not only from human demonstrations, but also from other sources such as satellites, aerial robots, and past demonstrations. By using a combination of different learning methods, they hope to create robots that can reason about the environment in the same way humans do and navigate any terrain with ease.
As Joydeep and his team continue to push the boundaries of what is possible in the field of robotics, we can only wait and see what he and his team will achieve next.
Collaborators on VRL-PAP include Kavan Singh Sikand, Sadegh Rabiee, Adam Uccello, Xuesu Xiao, and Garrett Warnell. Related ongoing research on terrain-adaptive navigation is being conducted in collaboration with Haresh Karnan, Elvin Yang, Daniel Farkash, Garrett Warnell, and Peter Stone. Stay tuned for further innovations in the space!